This post will discuss the potential risks of accepting a null hypothesis. This practice not only causes confusion in the understanding of biological responses but also leads to significant financial losses for producers. It is critical to be aware of these dangers and proceed with caution when interpreting experiment results.
The authors of this study [1] fed six pigs with ractopamine and six pigs with coconut oil, and because the statistical test used found no significant differences, they concluded that coconut oil can be used as a substitute for ractopamine. There were significant numerical differences in animal performance between groups, but these differences were not statistically significant, so they were ignored. Ractopamine is a compound with a chemical structure similar to epinephrine that has been shown to have anabolic properties that promote muscle growth in livestock. Coconut oil, in contrast, is a natural oil that has been used for centuries in cooking and has many potential health benefits. If you have a background in animal nutrition, you’re probably aware that although coconut oil may stimulate growth, it is unlikely to produce the same results as ractopamine. Using coconut oil as a replacement for ractopamine is a “earth is flat” type of assumption [2]. Suppose we hypothesize that the earth is a sphere and attempt to test this using a standard ruler. After multiple measurements, we will conclude that there is no effect (no curvature). Consequently, we might conclude that the earth is flat (P>0.05) or perhaps even square, depending on our initial assumptions. Making inferences with only 6 pigs out of 780 million pigs worldwide is analogous to using a ruler to measure the curvature of the Earth; we simply do not have the power to do so (the statistical power).
Assuming that coconut oil can be used to replace ractopamine is a difficult pill to swallow. However, in some cases, such as with low-protein diets, we may simply drink the Kool-Aid. In this study [3], the authors fed pigs diets with varying levels of protein and dietary fiber and concluded that diets higher in fiber and lower in protein than typical commercial diets have no negative effect on pig performance. The authors also noted that reducing protein led to better nitrogen (N) utilization and reduced ammonia emissions. The results showed a statistically significant decrease in N excretion, but no statistical differences in other parameters. Therefore, the authors accepted the hypothesis that reducing protein (or N) intake did not impact other variables. As shown in Figure 1, there was a 29% decrease in N excretion in this study, which was accounted for, but there was also a similar decrease in N retention, which was ignored because the p-value was not low enough.
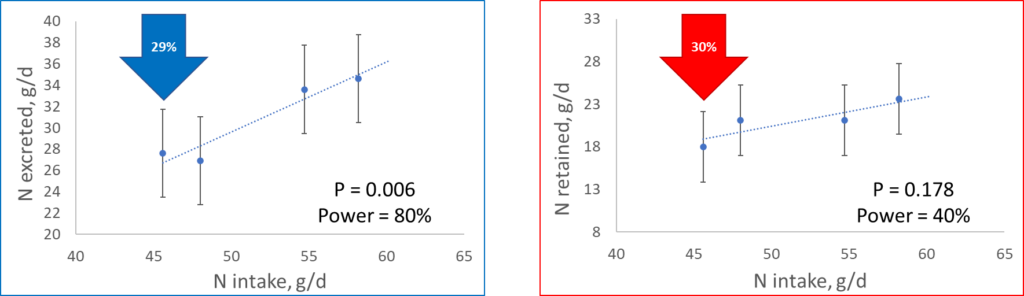
In this case, a 12.6 g reduction in N intake resulted in a 7 g reduction in N excretion but also a 5.6 g reduction in N retention (Table 1). As the calculations in Figure 2 shown, reducing 12.6 g of N in the diet saves $0.25 per pig per day but results in a $0.35 loss of body weight per pig per day. If we consider a 1200-head pig barn, the practice recommended by paper [3] would result in a loss of $120 per day.
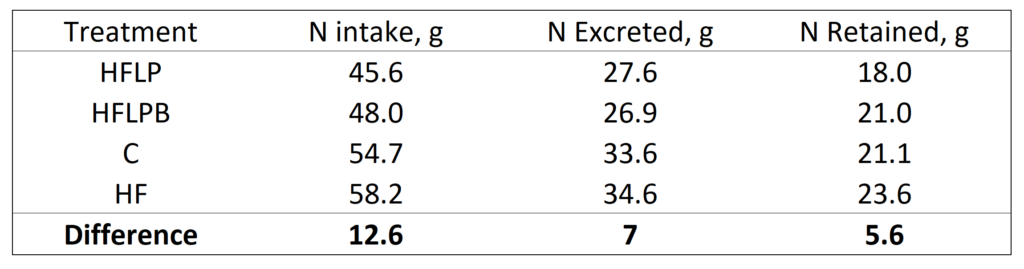
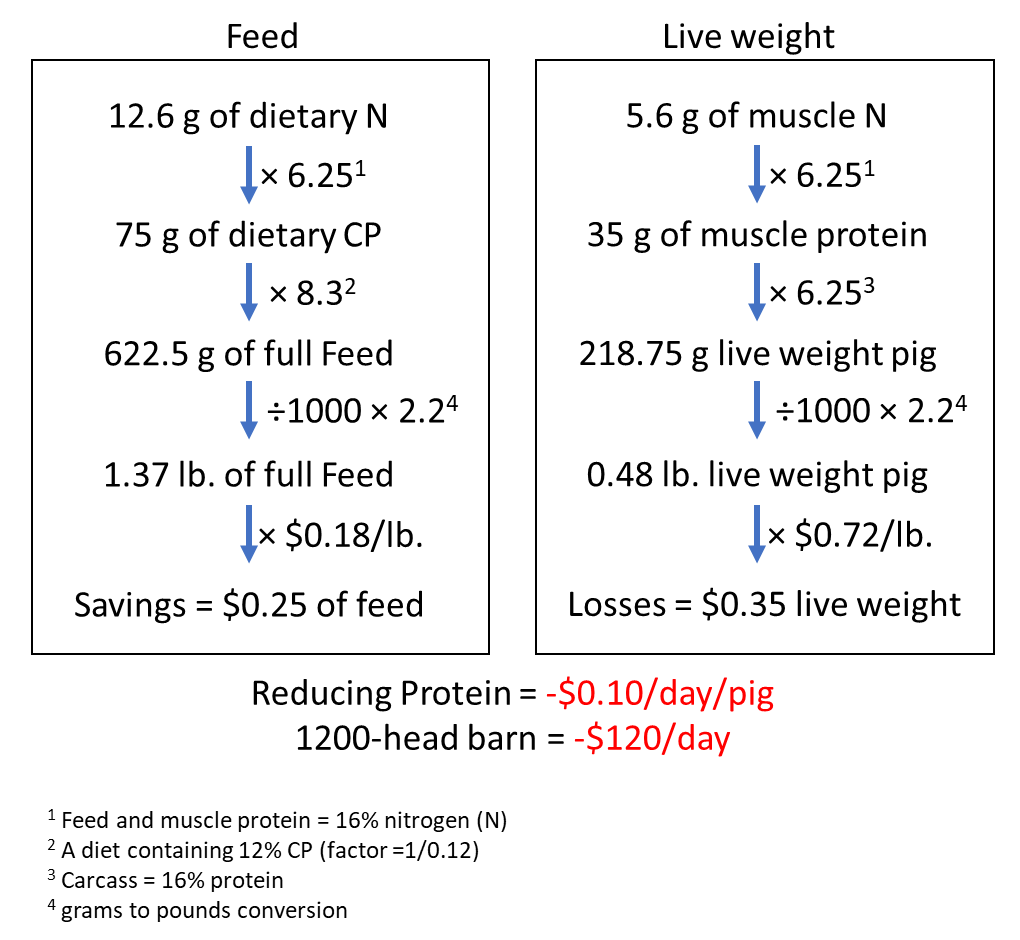
The aforementioned study used six animals per group. While this sample size may suffice to identify differences in variables like N excretion, it is inadequate for variables like N retention, where the sample size of six per group only provides 40% statistical power. Similar experiments in which the authors base their conclusions solely on a single significant variable (for example, measures of N excretion) while ignoring the rest of the data are common in the literature, especially when the sample size is small. I’m counting on you to spot studies like this on your own, there are plenty.
The results of studies with a small number of animals are simply unreliable. As illustrated in Video 1, which depicts two simulated populations, small sample sizes lead to unpredictable behavior of means and p-values. In this example, after drawing 50 samples from the population, the means and p-values of both groups stabilize. Video 1 provides an example showing that a sample size of less than 50 may not be enough to detect a 4% difference in performance.
Video 1. Two different populations were simulated (True mean A = 104; true mean B = 100; standard deviation for both groups = 13). At each iteration, one more observation was added to the sample. The P-values of a t-test are displayed.
There are certain scenarios where detecting differences requires only a small number of observations, particularly when the effect is relatively large. In the two simulated populations depicted in Video 2, ten or more observations are enough to detect statistically significant differences. In this case the difference between Treatment A and B is 15%.
Video 2. Two different populations were simulated (True mean A = 115; true mean B = 100; standard deviation for both groups = 13). At each iteration, one more observation was added to the sample. The P-values of a t-test are displayed.
In the same experiment, different variables may necessitate a different number of observations in order to confidently study the effects of the intervention (like in the example of reduced protein + fiber). Prior to conducting a study, it is recommended that the number of observations required to detect statistical differences in the variable with the smallest expected proportional change be determined. Other factors, such as the standard deviation and the statistical method used, should also be considered to estimate optimal sample size; these factors will be covered in future posts.
The authors drew their conclusions about reduced protein + fiber diets based on a selective analysis of low powered data. They stated: : “It is concluded that increasing the fibre content of the diet together with a decrease of the protein content reduced N excretion without jeopardizing performance of heavy pigs”. If you are a savvy entrepreneur, you can use the studies mentioned above to sell your own products to pig farmers. Is there anyone here who could use some dietary fiber? Coconut oil, perhaps.
Final remarks:
Rejecting some null hypotheses while accepting all others may get our papers published, especially if they align with current dogma; however, we may be harming farmers and society as a whole by decreasing the efficiency with which our food is produced. One common justification for continuing with the same practices (for example, recommending reduced-protein diets based on low-powered studies) is that if the industry is doing it, it must be profitable. In the preceding example with reduced protein diets + fiber, a producer can identify the $0.25 reduction in feed costs per pig per day, but most, if not all, producers do not have the ability to measure $0.10 losses per pig per day in opportunity costs. We as an industry can’t even count the pigs in our barns reliably [4], let alone account for losses of 10 cents per pig per day. Because these measurements are difficult, the industry relies on scientists. The problem is that scientists rely on p-values, which, as previously discussed, do not mean much, especially if the scientists do not know how to perform a power test. Indeed, some researchers advocate for the abolition of p-values, claiming that they give scientists a false sense of certainty [5].
Questions for the reader:
- Are you able to conduct a power test?
- What alternatives to p-values are there?
Thanks for reading, and I hope you found this post helpful! Your feedback was extremely helpful in the creation of this post. Please leave your thoughts and suggestions for future entries.
Christian R
References
1. Costa, C.V.S.d., et al., Substitution of ractopamine by safflower or coconut oil as an additive in finishing pig diets. Ciência Rural, 2020. 50.
2. Amrhein, V., F. Korner-Nievergelt, and T. Roth, The earth is flat (p> 0.05): significance thresholds and the crisis of unreplicable research. PeerJ, 2017. 5: p. e3544.
3. Galassi, G., et al., Effects of high fibre and low protein diets on performance, digestibility, nitrogen excretion and ammonia emission in the heavy pig. Animal Feed Science and Technology, 2010. 161(3-4): p. 140-148.
4. Tian, M., et al., Automated pig counting using deep learning. Computers and Electronics in Agriculture, 2019. 163: p. 104840.
5. Greenland, S., et al., Statistical tests, P values, confidence intervals, and power: a guide to misinterpretations. European journal of epidemiology, 2016. 31: p. 337-350.
3 thoughts on “Coconut oil and dietary fiber: the panacea of pig production”