In animal agriculture, a common practice involves conducting studies in research facilities and subsequently extrapolating their findings to broader populations. These studies are typically carried out by either an organization’s Research and Development Department or by external companies, which then use the study results to inform their strategies or product decisions. However, it’s worth noting that in numerous instances, these research teams exhibit deficiencies in their comprehension of the statistical methods they employ and a limited grasp of the dynamics within the various layers of the swine industry (i.e., pig> research barns> production barns).
As discussed in a previous post, a good example concerns the recommendation of using low-protein diets. In a traditional experiment aimed at assessing the effect of reducing dietary crude protein while supplementing with crystalline amino acids or other ingredients, the common conclusion is that “reduced protein diets cause a decrease in nitrogen excretion with no effect on animal performance.” However, it’s essential to recognize that this conclusion is opinion-based rather than scientific. In these experiments, it is typical to reduce dietary crude protein by 2 to 3% and supplement it with additives. However, to detect whether this reduction in dietary crude protein has a significant impact on pig growth or mortality, statistical power calculations indicate that more than 100,000 animals per group may be needed. Consequently, these types of experiments often lack the resolution or resources necessary to detect the effects of their interventions, leading to the erroneous conclusion that the intervention has no effect. Some companies and research groups go even further, advising producers to reduce protein, feed intake, energy, or other nutrients by 0.5 to 3% without adding any additives to achieve optimal “economic output”. In these scenarios, other effects are often completely disregarded. As explained further below, reducing nutrient intake does indeed influence pig survival, but this is frequently overlooked due to a limited understanding of statistical methods and animal biology, resulting in economic losses and welfare concerns.
The examples mentioned above can be misleading for producers and nutritionists due to a common misunderstanding of p-values. In experimental research, the absence of statistical significance (P > 0.05) does not necessarily indicate the absence of real effects. From a practical perspective, p-values have limitations and often reflect an evaluation of the methods used rather than a true biological response. When a study fails to detect statistical differences, it typically signifies that the methods employed may not have been suitable for detecting those differences. This could be due to a small sample size, inappropriate statistical techniques, or, more commonly, a combination of both factors.
It is possible to conclude that an intervention has no effects on pig “performance” if a power test is conducted before the experiment. However, another prevalent issue in these studies is the poor definition of “performance.” Often, power tests are exclusively performed on growth-related variables, while other crucial factors such as mortality, longevity, and health-related variables are overlooked.
To accurately conclude that an experiment did not affect “performance,” power tests must encompass ALL variables linked to pig performance, rather than focusing solely on growth-related parameters or the variable that the researcher deems “important.” As elaborated upon below, this skewed approach has led to an increase in pig removal rates. These experiments, concentrating solely on growth, neglected other critical responses, obtained p-values supporting their hypotheses, and erroneously applied the data from research barns to production sites. What appears to be transpiring is that certain effects, not initially evident in small sample studies, manifest their consequences in larger populations, contributing to the elevated pig mortality rates observed across the swine industry.
Thus, we inadvertently increase pig mortality due to a poor understanding of statistical methods, leading us to overlook certain effects because the studies lacked the capacity or resolution to detect them in the first place. Consequently, we end up extrapolating flawed ‘science’ to larger populations, causing considerable harm. Additionally, when a non-significant p-value (P > 0.05) conceals the mortality effect, survivor bias becomes more pronounced. In other words, if our interventions unintentionally kill the weakest animals, our practices may appear effective when we analyze data from the survivors, who are typically the heavier and more robust animals. Consequently, if we ‘correct’ the data by ignoring mortality, we mistakenly perceive ourselves as an industry that produces [surviving] pigs very efficiently. However, this false sense of efficiency is fundamentally rooted in a poor understanding of the system.
A Short Comment On Statistical Models
In the previous examples of experiments, the commonly employed methodology often utilizes ANOVA. However, this method is often inappropriate because animal responses, such as growth, survival, and health, are continuous variables (numeric), just like nutrient supply factors such as dietary protein, amino acids, and energy. In such cases, ANOVA becomes inadequate for reasons explained in a previous post, rendering the conclusions drawn from this method invalid.
Furthermore, complex systems like pig populations often exhibit non-linear dynamics. Consequently, linear models (e.g., linear and quadratic) are frequently unsuitable for studying these intricate dynamics. It is imperative to employ non-linear models to understand these systems better. However, it’s worth noting that non-linear models often yield p-values that are not meaningful in many cases.
If we are committed to a deeper understanding of these systems, we must move beyond a reliance on p-values. Alternatively, if p-values are to be used, they should be interpreted with a profound understanding of their limitations.
Assumption-Based vs. Data-Based Conclusions
In livestock experiments, it is often assumed that measuring the effect of an intervention on growth is sufficient to gauge the intervention’s impact on various aspects of animal performance. This assumption is grounded in the concept of the hierarchy of nutrient use, a mental model established over 70 years ago. According to this model, various bodily functions and systems follow a hierarchy, with some being prioritized over others in terms of their importance for an animal’s survival.
In this hierarchy, functions such as maintenance, reproduction, lean tissue deposition, and adipose tissue deposition are arranged in a specific order. More critical functions, like those associated with the immune system, digestive system, and reproductive system, take precedence over less vital aspects such as lean tissue deposition. Therefore, the underlying assumption is that when an animal achieves maximum growth, it signifies that the more crucial functions necessary for its survival have already been adequately addressed.
However, research in ecology and related fields paints a different picture. As highlighted in my recent article [1], studies on wild boar have revealed a distinct prioritization in their allocation of resources—one that places lean tissue deposition above other functions such as the immune system, digestive system, and reproduction (termed maintenance by the hierarchy of nutrient use model). This preference for lean tissue appears to enhance their survival, particularly in the short term.
Pigs, like many other animals, employ two primary strategies to evade predation: hiding and running. Notably, wild boar has been observed concealing weaknesses, a behavior akin to pigs in commercial barns concealing lameness. This strategy suggests that these animals prioritize lean tissue deposition to maintain a healthy appearance, ultimately reducing their vulnerability to predators. Consequently, when nutrient availability decreases, it seems that animals opt to compromise systems that have less visible effects on their physical appearance—systems related to long-term survival—while preserving lean tissue for mobility, aiding in foraging, and evading predators, thus maximizing short-term survival. Thus, when feeding animals to maximize lean tissue deposition (growth), it appears that these levels fall short in optimizing functions crucial for long-term survival. These functions include aspects of the immune and digestive systems, among others (for further details, refer to [1]).
From a metabolic point of view, these phenomena can be explained as follows: after animals have met their amino acid and protein requirements to achieve maximum growth, amino acid catabolism occurs. This catabolism process results in the biosynthesis of various biomolecules that enhance functions beyond growth, including those related to health and survival.
This perspective suggests that when animals achieve maximum growth, other functions are not optimized. The situation worsens when we attempt to feed animals at dietary nutrient levels even below the threshold for maximum growth, using methods that do not allow to measure this effect, as seen in the previous example with low protein diets or strategies aimed at maximizing ‘economic output.’ As discussed in the reference below [1], optimal growth does not necessarily align with optimal survival. From this perspective, it is not surprising to observe high mortality and removal rates.
In addition, because animals seem to conceal their weaknesses, when we perform studies, animals may appear to be in their optimal body condition, but their systems may be compromised. In this scenario, neither the statistical methods nor the visual methods would allow us to detect the effects of the interventions. Keeping this in mind, we can change our statistical methods and biological measurements to better understand the effects of dietary intervention.
In summary, our limited grasp of statistical methods and biological responses can lead us to underestimate the potential impacts of interventions on animal health and survival, leading to economic losses and welfare issues. Within our studies involving pigs, we may encounter difficulties in uncovering the genuine influence of these interventions, largely due to our incomplete awareness of the underlying assumptions we employ. Frequently, we readily accept null hypotheses (or assume no effect when P > 0.05) and rely on the belief that measuring growth alone suffices, particularly when the pigs seem healthy. Nonetheless, it remains plausible that the authentic effects remain obscured by the complexities of statistical analyses and evolutionary mechanisms, which serve to mask animal vulnerabilities.
Recommendations:
- If someone is trying to sell you a product or a dietary strategy that promises substantial financial gains while claiming “no negative effects” on mortality, morbidity, or longevity, please share this post with them.
- Complexity is desirable when studying intricate systems like animals and populations. Please scrutinize oversimplified concepts, as they may provide convenient explanations but often fall short of accurately describing biological phenomena.
Thanks for reading, and I hope you found this post helpful!
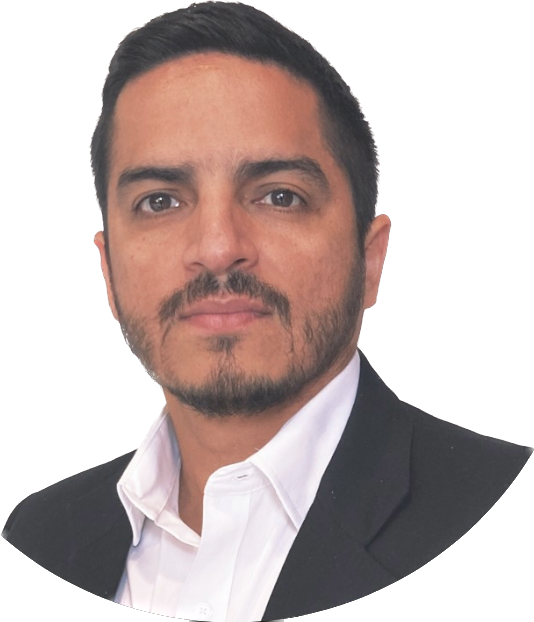
Christian Ramirez-Camba
Ph.D. Animal Science; M.S. Data Science