Big data has been touted as a solution for addressing numerous challenges across various domains, including the field of livestock production. Through the analysis of extensive big data sets containing historical production data, the goal is to unveil concealed patterns. This, in turn, could lay the groundwork for improvements in our production systems. However, a poor understanding of this tool can lead to precisely the opposite outcome. Because the results and analysis are based on a large number of animals, it may give us the illusion that our results must be accurate, hiding system inefficiencies, directing our efforts away from the main problems.
What is Big Data?
The definition of big data can vary depending on the context. To simplify the concept, we can consider big data as a database that programs like Microsoft Excel cannot process, either due to the sheer volume of data it contains or the speed at which this data needs to be queried. In such cases, Microsoft Excel would struggle to handle it, making our work more challenging. For the purposes of this post, I’ll further simplify the concept of big data as a dataset related to pig production that includes information on tens of thousands of animals.
Size Doesn’t Matter; It’s How Well You Can Use It
When it comes to data analysis, the quality of the data being analyzed is more important than the quantity. Having, for example, 40,000 animals in a study doesn’t automatically enhance the quality of the conclusions. In fact, a large number of animals can actually have a negative impact on data interpretation, particularly in systems with standardized practices, as it can create a false sense of certainty.
To illustrate this point, let’s consider an example from the banking industry. When financial institutions create models to assess the likelihood of various customer groups repaying loans on time, they intentionally lend to customers they know won’t be able to repay. Additionally, these institutions grant loans that are much higher or much lower than what their system recommends lending. This approach allows banks to study how the population’s responses vary. These observations are then used to obtain insights into the behavior of the system, thereby facilitating the development of predictive analytics models. These models, in turn, assist in making forecasts for similar customer groups in the future.
Now, returning to the field of animal production, particularly in swine production, historical data is typically rooted in highly standardized practices that leave little room for variation. The three main inputs in swine production—feed, genetics, and facilities—tend to operate within very narrow margins. Diets are often quite similar due to the nutrient requirements concept, and data on the responses of different genetic lines are often limited. Also, facilities are pretty much the same, focused on providing the same conditions. These three variables account for between 85% to 95% of the inputs of the system, which are basically static.
Therefore, if our historical data (big data) is based on identical animals following identical standardized practices, and our goal is to investigate the cause of a specific effect (such as piglet mortality), our conclusion is likely to be predominantly influenced by the variable with the most variability. In swine production, management is one of the variables that exhibits a high degree of fluctuation. Many studies have concluded that management is primarily responsible for issues such as pig mortality faced by the swine industry. However, when factors like management and other variables with a relatively minor impact are rigorously controlled, their impact on pig mortality is accordingly small.
It’s essential to recognize that other underlying causes may remain concealed due to a lack of observable responses. These latent responses cannot surface because we are not allowing the system to reveal them. This is analogous to accepting null hypotheses without the presence of associated p-values—it involves concluding an effect does not exist simply because we haven’t observed it. Nevertheless, our methods are not allowing the patterns to emerge. Conducting research within commercial facilities presents significant challenges, particularly because accountants often resist altering the established production flow with the mindset of “If it ain’t broke, don’t fix it.” However, can we consider a pig production system with over 30% pig removals as not already broken?
Data regarding the microbiome can also be considered as big data. In this case, instead of having production data from thousands of animals, we have thousands of chunks of data (e.g., bacteria) from a few animals. Although I will dedicate a different post to this topic, we are expecting that the microbiome will have the answers we are looking for. After all, the microbiome does fluctuate in our static system. We hope that one day it will give us the answers we are looking for, especially since it is becoming increasingly cost-effective.
This scenario reminds me of the streetlight effect, which is a type of observational bias that occurs when people only search for something where it is easiest to look, and it is linked to a well-known joke:
“A policeman sees a drunk man searching for something under a streetlight and asks what the drunk has lost. He says he lost his keys and they both look under the streetlight together. After a few minutes, the policeman asks if he is sure he lost them here, and the drunk replies, no, and that he lost them in the park. The policeman asks why he is searching here, and the drunk replies, ‘this is where the light is.'”
We may be looking for the keys by the streetlight. Don’t get me wrong, changes in the microbiome do lead to improvements in pig survival and other variables. However, most, if not all, interventions have positive effects, although they are relatively small compared to the scale of the problem. This is mainly because pigs are so undernourished that almost any intervention appears to yield positive results (which is also a topic for a future post, but for further reference, please see this previous post).
Statistical Power
In data analysis, what the data doesn’t reveal is just as crucial as what it does. It’s essential to examine our assumptions to understand what aspects of the system we might be neglecting. We must recognize that we cannot simply accept null hypotheses or conclude that a variable has no effect when we lack the appropriate number of observations (sample size) or when the system doesn’t exhibit a significant range of variation (effect size) for investigation. In essence, for any type of data analysis, including big data, we need to conduct power test calculations, which encompass considerations of sample size and effect size, before investing our time in analyzing data and drawing potentially misleading conclusions from low-quality data. Defining the effect size can be challenging, and it should be a primary concern for biologists when analyzing big data. The determination of effect size for a power test depends on expertise within the specific field of knowledge.
In summary, having thousands of observations doesn’t automatically enhance the validity of conclusions. To evaluate the quality of these conclusions, we must assess the quality of the data by examining which variables in the system were allowed to fluctuate and to what extent. This context is crucial for drawing meaningful conclusions. In production systems, certain variables do naturally fluctuate, and we often attribute the responses under study to these variables, such as the microbiome or management practices. However, we may not truly understand how to predict or modify these factors to influence the system’s behavior. These variables do not appear to be the primary cause of the problem; instead, they often show minor, weak correlations with limited impact on the system. It’s vital to comprehend the nature of the problem at hand so that we don’t end up searching for the keys at the streetlight.
Thanks for reading, and I hope you found this post helpful!
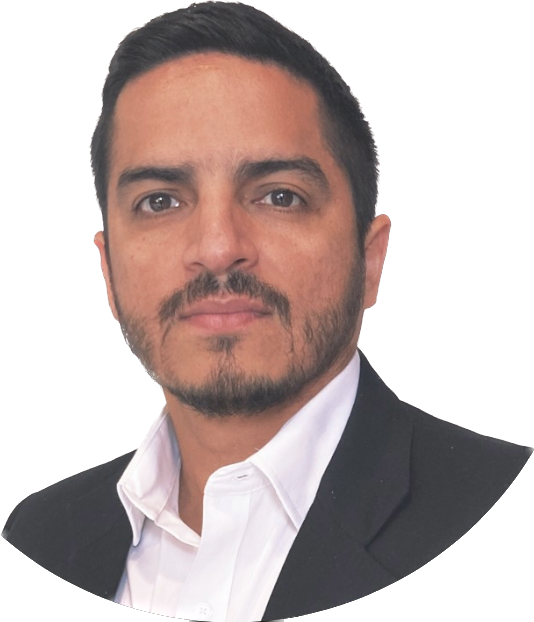
Christian Ramirez-Camba
Ph.D. Animal Science; M.S. Data Science